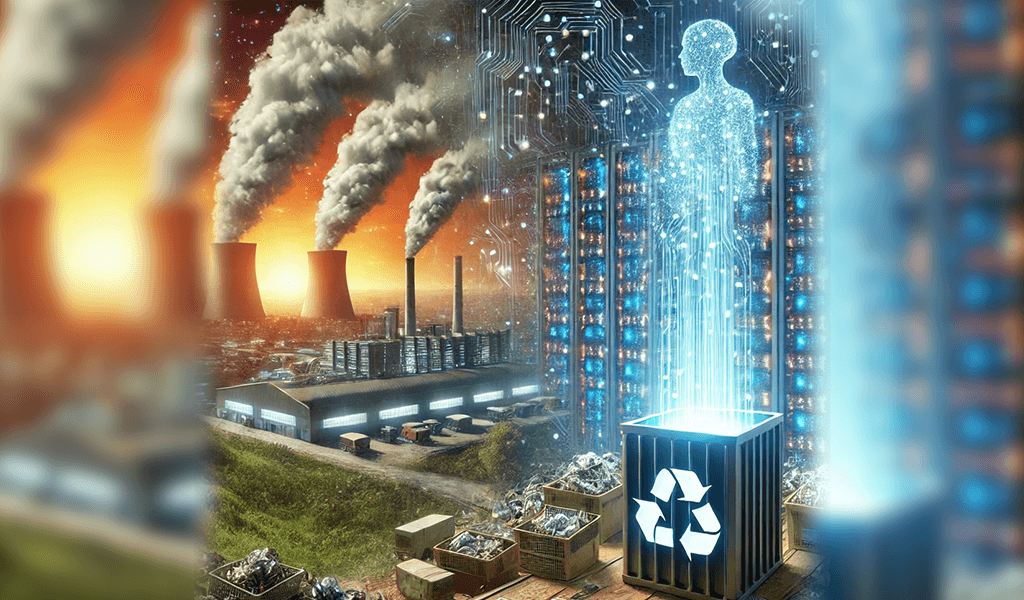
The Hidden Costs of AI Coding Development: Beyond the Obvious
1. The Environmental Toll of AI Development
Energy Consumption and Carbon Footprint
A 2019 study revealed that training a single large AI model can emit as much carbon dioxide as five cars over their entire lifetimes. The energy required to train models comes from data centers running 24/7, consuming electricity sourced from fossil fuels in many cases. As AI models grow larger, with billions of parameters, the energy requirements are set to increase.
E-Waste from Hardware Upgrades
AI development often necessitates cutting-edge hardware, such as GPUs and TPUs. The rapid obsolescence of such hardware leads to significant e-waste. Disposing of or recycling these components responsibly remains a challenge for many organizations.
2. The Financial Costs: Beyond Development
Infrastructure and Maintenance
AI systems require robust infrastructure, including high-speed internet, storage solutions, and cloud services. Maintaining this infrastructure can be costly, especially for small and medium-sized enterprises (SMEs). Furthermore, the ongoing costs of model retraining and updates add to the financial burden.
Skilled Workforce
AI development demands highly skilled professionals, including data scientists, machine learning engineers, and domain experts. The salaries for such roles are often exorbitant, given the demand-supply gap in the job market. Additionally, retaining such talent requires competitive compensation and benefits.
3. Social and Ethical Costs
Bias and Discrimination
AI systems are only as good as the data they are trained on. Biases present in training data can lead to discriminatory outcomes. For example, facial recognition systems have been criticized for their inability to accurately identify individuals with darker skin tones. Addressing these biases requires significant resources, from collecting diverse datasets to implementing fairness algorithms.
Job Displacement
As AI systems become more capable, they often replace human workers in various sectors, such as manufacturing, retail, and even white-collar jobs like data entry or customer support. This displacement creates economic challenges for workers, leading to societal unrest and calls for reskilling initiatives, which come at a cost.
4. Regulatory and Compliance Challenges
Compliance Costs
Meeting regulatory requirements, such as data privacy laws (e.g., GDPR) and ethical AI guidelines, involves substantial investments in legal expertise, audits, and system redesigns. Non-compliance can result in hefty fines and reputational damage.
Delays in Deployment
Navigating the regulatory environment can slow down the deployment of AI systems. These delays often translate into lost revenue opportunities, particularly in competitive markets where speed is crucial.
5. Long-term Costs of Maintenance and Scalability
Data Refreshing and Model Updates
AI models rely on up-to-date data to remain effective. Outdated models can produce inaccurate results, harming business operations. Regularly updating datasets and retraining models involve significant time and resource investments.
Scalability Challenges
As businesses grow, their AI systems need to scale accordingly. Scaling often requires migrating to more advanced cloud services or investing in new hardware, adding to the total cost of ownership.
6. The Psychological Cost on Developers
Burnout and Stress
The pressure to deliver high-performance AI systems within tight deadlines can lead to burnout among developers. This not only affects individual well-being but also impacts team productivity and innovation.
Ethical Dilemmas
Developers often face ethical dilemmas, such as whether to work on projects with potential for misuse. Navigating these challenges can be mentally taxing, adding to the hidden costs of AI development.
Addressing the Hidden Costs of AI Development
While the hidden costs of AI development are significant, they are not insurmountable. Businesses and developers can adopt several strategies to mitigate these costs:
- Adopt Green AI Practices: Opt for energy-efficient algorithms and hardware, and consider renewable energy sources for data centers.
- Invest in Fairness and Transparency: Implement tools to identify and mitigate biases, and ensure transparency in AI decision-making processes.
- Plan for Long-term Maintenance: Allocate budgets for ongoing model updates, data refreshing, and scalability requirements.
- Reskill the Workforce: Offer training programs to help workers adapt to roles that complement AI rather than compete with it.
- Prioritize Mental Health: Foster a healthy work environment with reasonable workloads and provide support for ethical decision-making.
- Engage with Policymakers: Stay informed about regulatory developments and actively participate in shaping policies that balance innovation with ethical considerations.
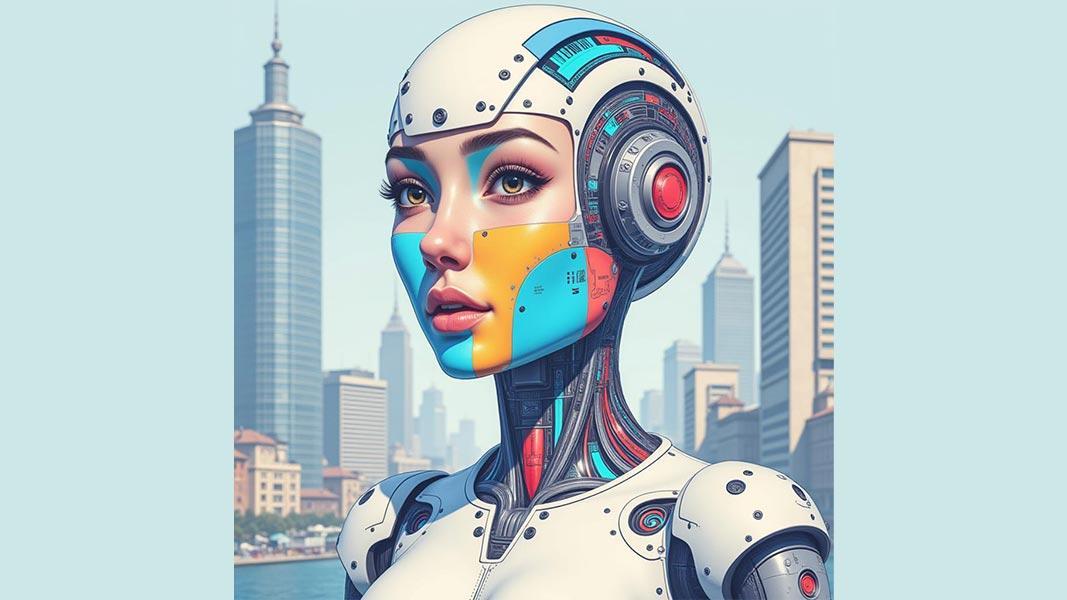
Revolutionizing Communication: AI Model Development to Enhance Language
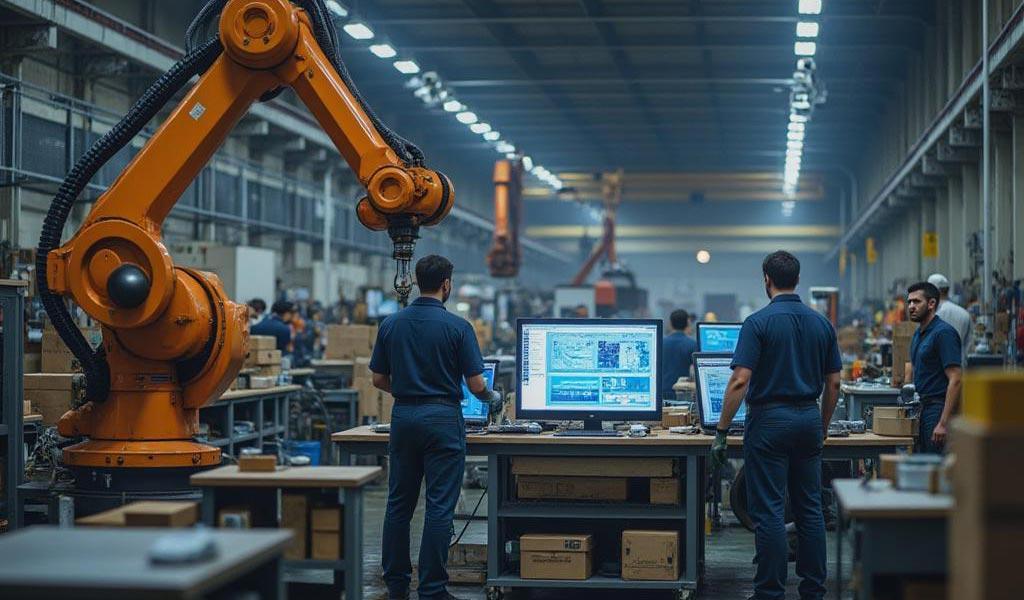
Revolutionizing Manufacturing: How AI Transforms Day-to-Day Operations
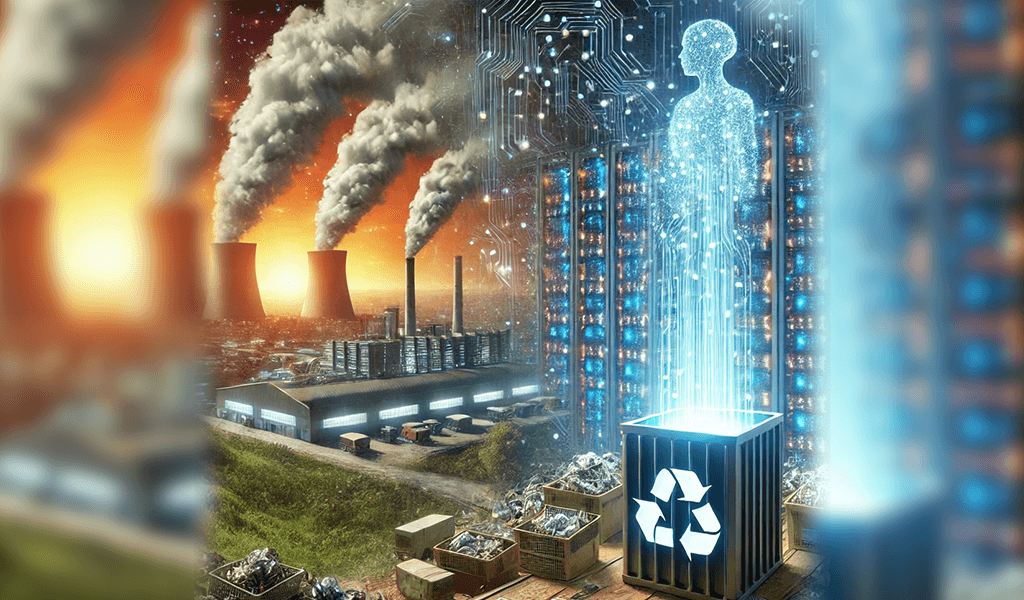